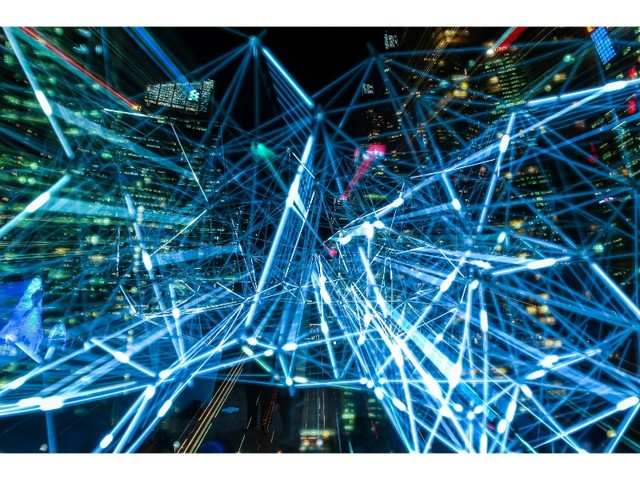
MIT researchers, including one of Indian-origin, have developed novel machine-learning techniques to improve the quality of life for patients by reducing toxic chemotherapy and radiotherapy dosing for an aggressive form of brain cancer.
Glioblastoma is a malignant tumour that appears in the brain or spinal cord, and the prognosis for adults is no more than five years.
Patients are generally administered maximum safe drug doses to shrink the tumour as much as possible, but they still remain at risk of debilitating side effects.
The new "self-learning" machine-learning technique could make the dosing regimen less toxic but still effective.
It looks at the treatment regimen currently in use, and finds an optimal treatment plan, with the lowest possible potency and frequency of doses that should still reduce tumour sizes to a degree comparable to that of traditional regimen, the researchers said.
"We kept the goal where we have to help patients by reducing tumour sizes but, at the same time, we want to make sure the quality of life -- the dosing toxicity -- doesn't lead to overwhelming sickness and harmful side effects," said Pratik Shah, principal investigator from the Massachusetts Institute of Technology (MIT) in Boston, US.
The findings will be presented at the 2018 Machine Learning for Healthcare conference at Stanford University in California, US.
In simulated trials of 50 patients, the model comprising of artificially intelligent "agents", designed treatment cycles that reduced the potency to a quarter or half of nearly all the doses while maintaining the same tumour-shrinking potential.
Many times, it skipped doses altogether, scheduling administrations only twice a year instead of monthly.
However, the researchers also had to make sure the model does not just dish out a maximum number and potency of doses. Whenever the model chooses to administer all full doses, it gets penalized, so instead it chooses fewer, smaller doses.
If all we want to do is reduce the mean tumour diameter, and let it take whatever actions it wants, it will administer drugs irresponsibly," Shah said.Instead, we need to reduce the harmful actions it takes to get to that outcome.
Glioblastoma is a malignant tumour that appears in the brain or spinal cord, and the prognosis for adults is no more than five years.
Patients are generally administered maximum safe drug doses to shrink the tumour as much as possible, but they still remain at risk of debilitating side effects.
The new "self-learning" machine-learning technique could make the dosing regimen less toxic but still effective.
It looks at the treatment regimen currently in use, and finds an optimal treatment plan, with the lowest possible potency and frequency of doses that should still reduce tumour sizes to a degree comparable to that of traditional regimen, the researchers said.
"We kept the goal where we have to help patients by reducing tumour sizes but, at the same time, we want to make sure the quality of life -- the dosing toxicity -- doesn't lead to overwhelming sickness and harmful side effects," said Pratik Shah, principal investigator from the Massachusetts Institute of Technology (MIT) in Boston, US.
The findings will be presented at the 2018 Machine Learning for Healthcare conference at Stanford University in California, US.
In simulated trials of 50 patients, the model comprising of artificially intelligent "agents", designed treatment cycles that reduced the potency to a quarter or half of nearly all the doses while maintaining the same tumour-shrinking potential.
Many times, it skipped doses altogether, scheduling administrations only twice a year instead of monthly.
However, the researchers also had to make sure the model does not just dish out a maximum number and potency of doses. Whenever the model chooses to administer all full doses, it gets penalized, so instead it chooses fewer, smaller doses.
If all we want to do is reduce the mean tumour diameter, and let it take whatever actions it wants, it will administer drugs irresponsibly," Shah said.Instead, we need to reduce the harmful actions it takes to get to that outcome.
No comments:
Post a Comment